Using Transfer Learning to Classify Breast Cancer Cells with Fluorescence Imaging
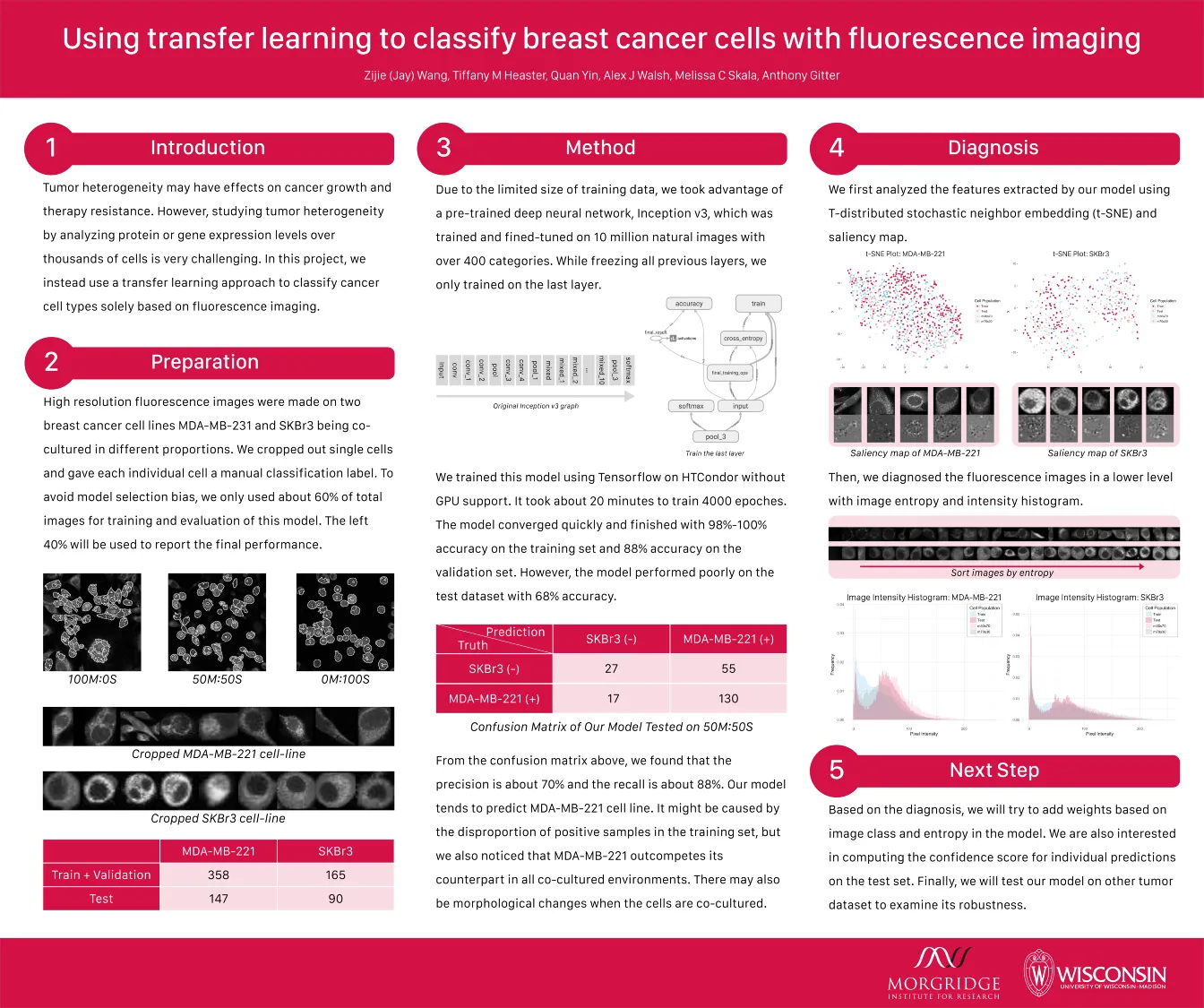
Abstract
Studying tumor heterogeneity by analyzing protein or gene expression levels over thousands of cells is very challenging. In this project, we instead use a transfer learning approach to classify cancer cell types solely based on fluorescence imaging. We used images of two types of breast cancer cell lines – MDA-MB-231 and SKBr3 – to partially retrain a deep convolutional neural network Inception v3, which was pre-trained on 10 million natural images with over 400 categories. We hypothesize features extracted from general pictures by a deep neural network are portable to classify breast cancer cell types. The ability to recognize distinct cell types within tumors would provide a powerful tool for analyzing clinical samples.
Citation
Using Transfer Learning to Classify Breast Cancer Cells with Fluorescence Imaging
@inproceedings{wang_using_poster_2018, title = {Using Transfer Learning to Classify Breast Cancer Cells with Fluorescence Imaging}, language = {en}, conference = {University of Wisconsin–Madison Undergraduate Symposium}, author = {Wang, Zijie J. and Heaster, Tiffany M. and Yin, Quan and Walsh, Alex J. and Skala, Melissa C. and Gitter, Anthony}, year = {2018} }