Dodrio: Exploring Transformer Models with Interactive Visualization
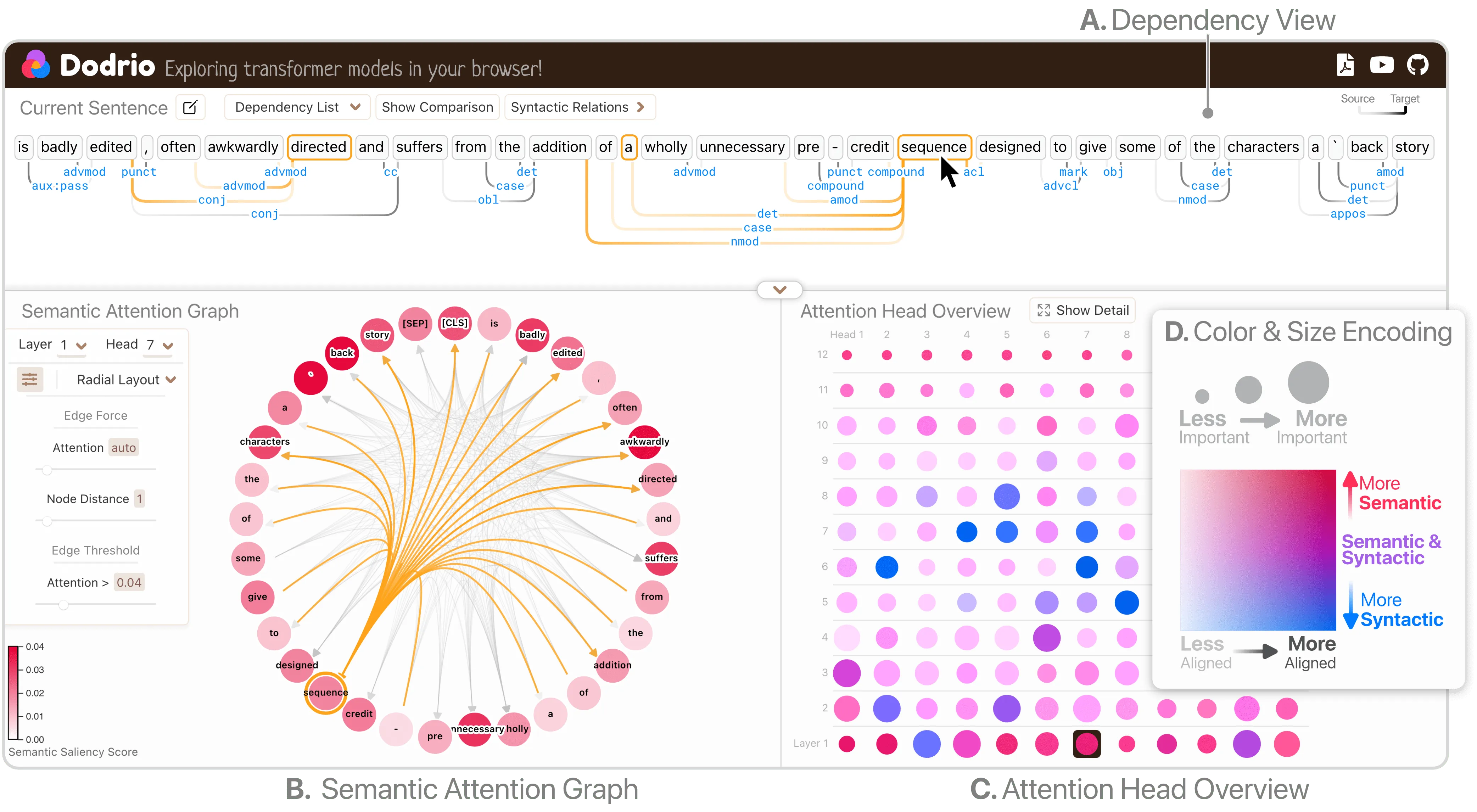
Demo Video
Abstract
Why do large pre-trained transformer-based models perform so well across a wide variety of NLP tasks? Recent research suggests the key may lie in multi-headed attention mechanism's ability to learn and represent linguistic information. Understanding how these models represent both syntactic and semantic knowledge is vital to investigate why they succeed and fail, what they have learned, and how they can improve. We present Dodrio, an open-source interactive visualization tool to help NLP researchers and practitioners analyze attention mechanisms in transformer-based models with linguistic knowledge. Dodrio tightly integrates an overview that summarizes the roles of different attention heads, and detailed views that help users compare attention weights with the syntactic structure and semantic information in the input text. To facilitate the visual comparison of attention weights and linguistic knowledge, Dodrio applies different graph visualization techniques to represent attention weights with longer input text. Case studies highlight how Dodrio provides insights into understanding the attention mechanism in transformer-based models. Dodrio is available at https://poloclub.github.io/dodrio/.
Citation
Dodrio: Exploring Transformer Models with Interactive Visualization
@inproceedings{wangDodrioExploringTransformer2021, title = {Dodrio: {{Exploring Transformer Models}} with {{Interactive Visualization}}}, author = {Wang, Zijie J. and Turko, Robert and Chau, Duen Horng}, shorttitle = {Dodrio}, booktitle = "Proceedings of the Joint Conference of the 59th Annual Meeting of the Association for Computational Linguistics and the 11th International Joint Conference on Natural Language Processing: System Demonstrations", year = {2021}, address = "Online", publisher = "Association for Computational Linguistics", pages = "132--141", url = {https://zijie.wang/papers/dodrio/} }